Why ERP Data Quality Matters During a CloudSuite Implementation
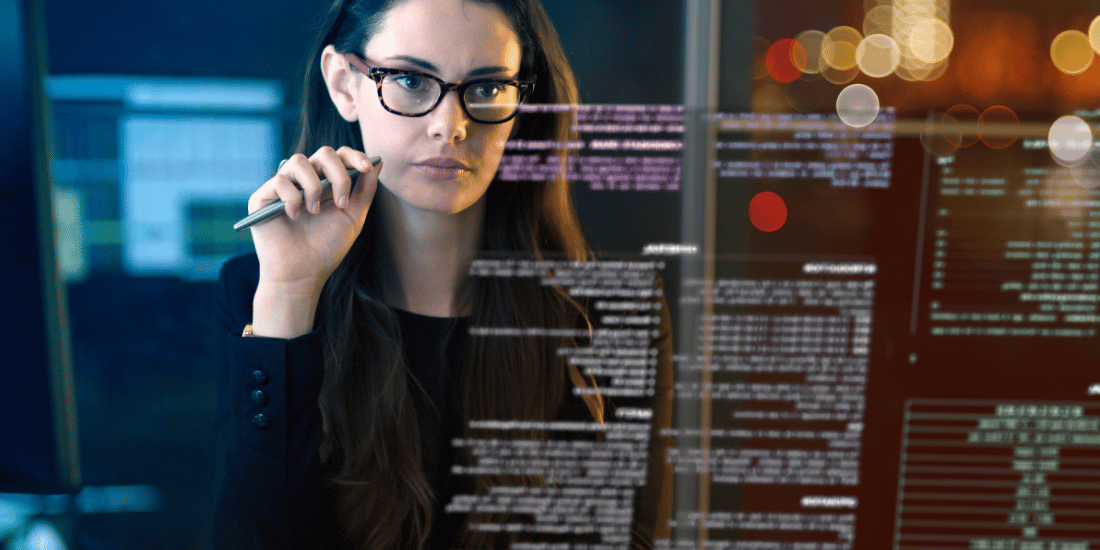
By: Melissa Olson August 8, 2023
“Dirty” or faulty data is bad news for business. In fact, Gartner research found that poor data quality costs businesses an average of $12.9 million a year. An earlier survey found that 60% of businesses don’t even measure the annual cost of poor quality data. If you believe you don’t have any dirty data, you probably haven’t found it yet.
Data quality is especially important during an ERP implementation. But there’s more to it than cost. Progressing an ERP implementation with poor quality data carries several risk factors. It can impact revenue and operational expenses, affect your company’s reputation, and lead to legal and regulatory issues.
If your ERP system holds inaccurate, incomplete, or inconsistent data, it becomes difficult to trust your reporting and analytics. In healthcare, for example, research suggests that poor data quality impacts staffing, decision making, and patient care. Improvement in data quality in healthcare could result in $42.1 million in savings.
Why ERP Data Quality Matters
Dirty data is structured and unstructured information that is faulty or contains errors. This might be duplicate or outdated information, data that is incomplete, or not mapped correctly in the database schema. Examples of dirty data include missing field values, incorrect formats (such as phone numbers), duplicate supplier records or, most commonly, values referencing legacy organizational structure.
Ensuring data quality during your ERP implementation requires planning, validating, cross-checking, and creating workflows to audit information. Dashboards, reports, and machine learning can also help with visibility and error handling. Ultimately these methods will help verify whether your data can answer relevant questions accurately.
If data quality issues are identified and not resolved during an ERP implementation, the implementation may fail. Plain and simple. That’s why it’s paramount that organizations assess and validate their data through the dimensions of data quality prior to an ERP implementation.
Avoiding Data Quality Issues During an ERP Implementation
Quality data can be defined as data that meets these five criteria:
- Complete: Complete data means there are no missing values, which typically result from data entry errors or collection problems. Ask yourself, “Is all the data my organization will need available?” and “Can I use all the data to answer the questions we’ll have?” If the answer to both questions is yes, your data is complete.
- Consistent: Consistent data is void of duplicative or inaccurate information. Inconsistencies in data can occur when information is transferred from legacy systems during an ERP implementation. Watch for differing formats and column names; for example, is address information stored in one column or is it broken out into several? Does your new ERP system have a place to receive values stored in custom fields and tables? If not, will you configure your new system to keep them, or will you retain them in an archival solution?
- Timely: Real-time data enables informed decision-making. The data in your ERP must be current. Can your data be used for real-time reporting? You can check to see if fields were set as mandatory or for auto-completion. If your data isn’t up to date, it’s important to document the schedule in which it will update and inform stakeholders accordingly. The most important aspect regarding timely data is that you’re aware of its age.
- Accurate: Your dataset might have been accurate several months (or years) ago, but data changes rapidly and needs to make sense within a given context. Does an email address belong to an actual domain? Or can a bank account or tax-exempt number be verified online? Take measures to verify the accuracy of the information recorded in your databases.
- Valid: Does the data meet your organization’s requirements, that is, does it satisfy specific conditions? For instance, setting a credit limit for a client might require maximum values, or a range based on a client group, to be agreed upon. Ensure that the data stored in your systems adheres to your specific conditions.
Once you’ve reviewed your data through the various dimensions of quality, you can develop a plan for addressing them prior to your ERP implementation.
Creating an ERP Data Quality Implementation Plan
Gartner recently identified 12 actions to improve data quality, gathered into four categories to keep in mind when evaluating your data’s integrity:
- Focus on the right things in setting strong foundations
- Apply data quality accountability
- Establish ‘’fit for purpose’’ data quality
- Integrate data quality into corporate culture
In addition, the following steps can help avoid data quality issues during an ERP implementation:
- Clarify what data is needed: According to Gartner, not all data is equally important. The research and consulting firm recommends focusing on the data with the most influence on business outcomes. In a cloud migration, the less transactional data, the smoother the conversion process. If you bring over every piece of data, on the basis of ‘’just in case,’’ the project becomes more complex. Start by focusing on high-priority data and work from there. You may want to delete segments of your data; however, there might be regulatory requirements that require you to keep it. At this point, it’s recommended that you meet with key stakeholders to obtain agreement on validation rules for standardizing and cleaning your data. For example, create filters to narrow down the amount of detail provided, say, in job function or level of education.
- Clean your data: As you identify how much data you need to transfer, consider the process required for cleaning it. This should help in assessing the amount of resources required for cleaning it before transitioning to a cloud-based system. Data cleansing can be automated using tools such as OpenRefine, but the data must still be reviewed to identify inconsistencies. During the cleaning process, data verification ensures that information is available, accessible, complete, and in the proper format. Best practices for cleaning data include validating accuracy, managing duplicates, and appending missing data; RPI has more than two decades of experience in helping organizations clean and transfer their data. In difficult scenarios, it isn’t possible to clean the data before migration. In cases where the time and effort are too high to update the legacy system, or the structure of the legacy system will not allow for the transformed values, thoughtfully build cross mapping into the conversion files. You will have to continuously update cross mapped data as day-to-day operations create more data in the old format.
- Ensure data validity: The validation process can begin with completing missing values and eliminating duplicate records. Getting rid of duplicates is especially critical during an ERP implementation. There are exceptions, though–some organizations will use the same vendor bank account, for example. You’ll want to ensure your data is “fit for purpose,” or, in other words, capable of being used to meet your objectives or service levels. To do so, Gartner recommends performing data profiling—that is, “examining data from an existing source and summarizing information” about it—as well as data monitoring, for example, by using a data quality dashboard. Both will help you validate current data gaps and challenges.
- Conduct data analysis: Once you’ve validated your data, analyze it within its greater context. Consider the measures taken for capturing this data and what’s being done with it. This exercise can help you optimize performance by delivering a comprehensive “state of the business,” which will allow you to make more informed decisions. This will also help you determine which of your current reports are rendered irrelevant, not to be redeveloped during implementation. For example, you can create more efficient processes, cut redundancies, and adjust to shifting market conditions. RPI can guide your company with this valuable analysis based on the findings from other organizations in the same industry with similar operational goals.
- Integrate data quality into your culture: Ensuring ERP data quality is not a one-time process but requires ongoing evaluation to ensure trustworthy data. Identifying and taking steps to improve issues around data quality will help optimize business performance and outcomes. Consider knowledge sharing and collaboration across your organization. After an ERP implementation, regular checks should be conducted across your system. Use solutions that automate data cleansing and streamline your processes and consider a post-implementation audit in the first few months. Better yet, consult with an expert firm such as RPI to help your organization ensure data quality as you implement your ERP. We can help you develop a system that meets the five criteria for data quality mentioned above.
Ensuring ERP Data Quality
With over two decades of experience, RPI Consultants is the perfect ERP implementation partner for your Infor CloudSuite migration. We’ve partnered with hundreds of clients to provide tools and strategies for verifying and preparing data prior to kicking off their implementations. This is an equally important activity for organizations new to Infor and as well as those upgrading from Infor’s Lawson S3 ERP.
To learn more about how we can help you ensure trustworthy ERP data quality, contact us below.
Follow us online for faster access to announcements, knowledge base updates, and upcoming events!